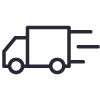
lieferbar, ca. 7-10 Tage
77,80 €
Preisangaben inkl. MwSt. Abhängig von der Lieferadresse kann die MwSt. an der Kasse variieren. Weitere Informationen
Topseller & Empfehlungen für Sie
Topseller & Empfehlungen für Sie
Ihre zuletzt angesehenen Produkte
Ihre Empfehlung
Mast
Understanding and Predicting Demand Through Visual Characteristics and Neural Networks
ISBN 978-3-339-13460-8
*
Diese Pflichtangaben werden zur erfolgreichen Verarbeitung Ihrer Weiterempfehlung benötigt.
Rezensionen